In what follows there is the list of my publications, chronologically ordered. Colors refers to works related to Mammography, Prostate, Brain, Other research publications, Educational papers.
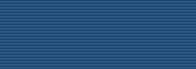
Journals
- [CMIG 2025]
U.M.Lal-Trehan Estrada, S.A.Sheth, A.Oliver, X.Lladó, and L.Giancardo.
Encoding 3D information in 2D feature maps for brain CT-Angiography.
Computerized Medical Imaging and Graphics, to appear, 2025.
[IF 5.4, Q1(18/204) RNMI]
- [UMB 2025]
M.Regalado, N.Carreras, C.Mata, A.Oliver, X.Lladó, and T.Agut.
Automatic segmentation of Sylvian fissure in brain ultrasound images of preterm infants using deep learning models.
Ultrasound in Medicine & Biology, 51(3), pp 543-550, 2025.
[IF 2.4, Q2(79/204) RNMI]
- [CMIG 2024]
C.Yalcin, V.Abramova, M.Terceño, A.Oliver, Y.Silva, and X.Lladó.
Hematoma expansion prediction in intracerebral hemorrhage patients by using synthesized CT images in an end-to-end deep learning framework.
Computerized Medical Imaging and Graphics, 117, id 102430, 2024.
[IF 5.4, Q1(18/204) RNMI]
- [CBM 2024]
A.Clèrigues, S.Valverde, A.Oliver, X.Lladó, and ADNI.
Improving brain atrophy quantification with deep learning from automated labels using tissue similarity priors.
Computers in Biology and Medicine, 179, id 108811, 2024.
[IF 7.0, Q1(18/169) CSIA]
- [ASc 2024]
V.Abramova, A.Oliver, J.Salvi, M.Terceño, Y.Silva, and X.Lladó.
An end-to-end deep learning framework for predicting hematoma expansion in hemorrhagic stroke patients from CT images.
Applied Sciences, 14(7), 2708, 2024.
[IF 2.5, Q1(44/179) EM]
- [iScience 2024]
U.M.Lal-Trehan Estrada, A.Oliver, S.A.Sheth, X.Lladó, and L.Giancardo.
Strategies to combine 3D vasculature and brain CTA with deep neural networks: application to LVO.
iScience, 27(2), 108881, 2024.
[IF 4.6, Q1(19/134) MS]
- [JMRI 2024]
Ll.Coll, D.Pareto, P.Carbonell-Mirabent, Á.Cobo-Calvo, G.Arrambide, A.Vidal-Jordana, M.Comabella, J.Castilló, B.Rodríguez-Acebedo, A.Zabalza, I.Galán, L.Midaglia, C.Nos, C.Auger, M.Alberich, J.Río, J.Sastre-Garriga, A.Oliver, X.Montalban, À.Rovira, M.Tintoré, X.Lladó, and C.Tur.
Global and regional deep learning models for multiple sclerosis stratification from MRI.
Journal of Magnetic Resonance Imaging, 60(1), pp 258-267, 2024.
[IF 3.3, Q1(42/204) RNMMI]
- [NI-Cl 2023]
Ll.Coll, D.Pareto, P.Carbonell-Mirabent, Á.Cobo-Calvo, G.Arrambide, A.Vidal-Jordana, M.Comabella, J.Castilló, B.Rodríguez-Acebedo, A.Zabalza, I.Galán, L.Midaglia, C.Nos, A.Salerno, C.Auger, M.Alberich, J.Río, J.Sastre-Garriga, A.Oliver, X.Montalban, À.Rovira, M.Tintoré, X.Lladó, and C.Tur.
Deciphering multiple sclerosis disability with deep learning attention maps on clinical MRI.
Neuroimage: Clinical, 38, art 103376, 2023.
[IF 3.4, Q2(4/15) NI]
- [CMIG 2023]
A.Clèrigues, S.Valverde, J.Salvi, A.Oliver, and X.Lladó.
Minimizing the effect of white matter lesions on deep learning based tissue segmentation from brain volumetry.
Computerized Medical Imaging and Graphics, 103, id 102157, 2023.
[IF 5.4, Q1(18/204) RNMMI]
- [MVA 2023]
H.Mahmood, Y.Diez, A.Oliver, J.Salvi, and X.Lladó.
Motion-region annotation for complex videos via label propagation across occluders.
Machine Vision and Applications, 34, art 2, 2023.
[IF 2.4, Q2(14/32) CSC]
- [FNSc 2022]
M.Salem, M.A.Ryan, A.Oliver, K.F.Hussain, and X.Lladó.
Improving the detection of new lesion sclerosis with a cascaded 3D fully convolutional neural network approach.
Frontiers in Neuroscience, id 1007619, 2022.
[IF 4.3, Q2(94/272) N]
- [FNSc 2022]
L.Valencia, A.Clèrigues, S.Valverde, M.Salem, A.Oliver, À.Rovira, and X.Lladó.
Evaluating the use of synthetic T1-w images in new T2 lesion detection in multiple sclerosis.
Frontiers in Neuroscience, id 954662, 2022.
[IF 4.3, Q2(94/272) N]
- [CP 2022]
C.Mata, P.Walker, A.Oliver, J.Martí, and A.Lalande.
Usefulness of collaborative work in the evaluation of prostate cancer from MRI.
MDPI Clinics and Practice, 12, pp 350-362, 2022.
[IF 2.3, Q2(128/322) MGI]
- [MELBA 2022] R.Mehta et al. QU-BraTS: MICCAI BraTS challenge on quantifying uncertainty in brain tumor segmentation - Analysis of ranking scores and benchmarking results. Journal of Machine Learning for Biomedical Imaging, id 026, pp 1-54, 2022.
- [MS 2022]
À.Rovira, J.F.Corral, C.Auger, S.Valverde, À.Vidal-Jordana, A.Oliver, A.Barros, Y.K.Ng-Wong, M.Tintoré, D.Pareto, F.X.Aymerich, X.Montalban, X.Lladó, and J.Alonso.
Assessment of automatic decision-support systems for detecting active T2 lesions in multiple sclerosis patients.
Multiple Sclerosis Journal, 28(8), pp 1209-1218, 2022.
[IF 5.8, Q1(31/212) CN]
- [JMRI 2021]
S.Valverde, Ll.Coll, L.Valencia, A.Clèrigues, A.Oliver, J.C.Vilanova, Ll.Ramió-Torrentà, À.Rovira, and X.Lladó.
Assessing the accuracy and reproducibility of PARIETAL: a deep learning brain extraction algorithm.
Journal of Magnetic Resonance Imaging, 59(6), pp 1991-2000, 2024.
[IF 5.119, Q1(33/136) RNMMI]
- [CMIG 2021]
V.Abramova, A.Clèrigues, A.Quiles, D.Garcia Figueredo, Y.Silva, S.Pedraza, A.Oliver, and X.Lladó.
Hemorrhagic stroke lesion segmentation using a 3D U-Net with squeeze-and-excitation blocks.
Computerized Medical Imaging and Graphics, 90, art 101908, 2021.
[IF 7.422, Q1(14/136) RNMMI]
- [FNS 2021]
K.Kushibar, M.Salem, S.Valverde, À.Rovira, J.Salvi, A.Oliver, and X.Lladó.
Transductive transfer learning for domain adaptation in brain magnetic resonance image segmentation.
Frontiers in Neuroscience, id 608808, 2021.
[IF 5.152, Q2(87/274) N]
- [NINF 2021]
J.Bernal, S.Valverde, K.Kushibar, M.Cabezas, A.Oliver, X.Lladó, and the Alzheimer's Disease Neuroimaging Initiative.
Generating longitudinal atrophy evaluation datasets on brain magnetic resonance images.
Neuroinformatics, 19(3), pp 477-492, 2021.
[IF 4.085, Q2(38/112) CSIA]
- [ASc 2021]
E.E.Carvajal-Camelo, J.Bernal, A.Oliver, X.Lladó, M.Trujillo, and the Alzheimer's Disease Neuroimaging Initiative.
Evaluating the effect of intensity standardisation on longitudinal whole brain atrophy quantification ion brain magnetic resonance images.
Applied Sciences, 11(4), art 1773, 2021.
[IF 2.679, Q2(38/91) EM]
- [NI-Cl 2020]
S.González-Villà, A.Oliver, Y.Huo, X.Lladó, and B.A.Landman.
A fully automated pipeline for brain structure segmentation in multiple sclerosis.
NeuroImage: Clinical, 27, art 102306, 2020.
[IF 4.881, Q2(4/14) NI]
- [CMPB 2020]
A.Clèrigues, S.Valverde, J.Bernal, J.Freixenet, A.Oliver, and X.Lladó.
Acute and sub-acute stroke lesion segmentation from multimodal MRI.
Computer Methods and Programs in Biomedicine, 194, art 105521, 2020.
[IF 5.428, Q1(13/110) CSTM]
- [NI-Cl 2020]
M.Rakić, M.Cabezas, K.Kushibar, A.Oliver, and X.Lladó.
Improving the detection of autism spectrum disorder by combining structural and functional MRI information.
NeuroImage: Clinical, 25, art 102181, 2020.
[IF 4.881, Q2(4/14) NI]
- [NI-Cl 2020]
M.Salem, S.Valverde, M.Cabezas, D.Pareto, A.Oliver, J.Salvi, À.Rovira, and X.Lladó.
A fully convolutional neural network for new T2-w lesion detection in multiple sclerosis.
NeuroImage: Clinical, 25, art 102149, 2020.
[IF 4.881, Q2(4/14) NI]
- [CBM 2019]
A.Clèrigues, S.Valverde, J.Bernal, J.Freixenet, A.Oliver, and X.Lladó.
Acute ischemic stroke lesion core segmentation in CT perfusion images using fully convolutional neural networks.
Computers in Biology and Medicine, 115, art 103487, 2019.
[IF 3.434, Q1(8/59) MCB]
- [ACCESS 2019]
J.Bernal, K.Kushibar, M.Cabezas, S.Valverde, A.Oliver, and X.Lladó.
Quantitative analysis of patch-based fully convolutional neural networks for tissue segmentation on brain magnetic resonance imaging.
IEEE Access, 7(1), pp 89986-90002, 2019.
[IF 3.745, Q1(35/156) CSIS]
- [SRep 2019]
K.Kushibar, S.Valverde, S.González-Villà, J.Bernal, M.Cabezas, A.Oliver, and X.Lladó.
Supervised domain adaptation for automatic sub-cortical brain structure segmentation with minimal user interaction.
Scientific Reports, 9, art 6742, 2019.
[IF 3.998, Q1(17/71) MS]
- [MIA 2019]
E.García, Y.Diez, O.Diaz, X.Lladó, A.Gubern-Mérida, R.Martí, J.Martí, and A.Oliver.
Breast MRI and X-ray mammography registration using gradient values.
Medical Image Analysis, 54, pp 76-87, 2019.
[IF 11.148, Q1(4/136) CSAI]
- [ACCESS 2019]
M.Salem, S.Valverde, M.Cabezas, D.Pareto, A.Oliver, J.Salvi, À.Rovira, and X.Lladó.
Multiple sclerosis lesion synthesis in MRI using an encoder-decoder U-Net.
IEEE Access, 7(1), pp 25171-25184, 2019.
[IF 3.745, Q1(35/156) CSIS]
- [NI-Cl 2019]
S.González-Villà, A.Oliver, Y.Huo, X.Lladó, and B.A.Landman.
Brain structure segmentation in the presence of multiple sclerosis lesions.
Neuroimage: Clinical, 22, art 101709, 2019.
[IF 4.350, Q2(4/14) NI]
- [NI-Cl 2019]
S.Valverde, M.Salem, M.Cabezas, D.Pareto, J.C.Vilanova, Ll.Ramió-Torrentà, À.Rovira, J.Salvi, A.Oliver, and X.Lladó.
One-shot domain adaptation in multiple sclerosis lesion segmentation using convolutional neural networks.
Neuroimage: Clinical, 21, art 101638, 2019.
[IF 4.350, Q2(4/14) NI]
- [AIIM 2019]
J.Bernal, K.Kushibar, D.S.Asfaw, S.Valverde, A.Oliver, R.Martí, and X.Lladó.
Deep convolutional neural networks for brain image analysis on magnetic resonance imaging: a review.
Artificial Intelligence in Medicine, 95, pp 64-81, 2019.
[IF 4.383, Q1(30/156) CSAI]
- [MIA 2018]
K.Kushibar, S.Valverde, S.González-Villà, J.Bernal, M.Cabezas, A.Oliver, and X.Lladó.
Automated sub-cortical brain structure segmentation combining spatial and deep convolutional features.
Medical Image Analysis, 48, pp 177-186, 2018.
[IF 8.880, Q1(5/133) CSAI]
- [TMI 2018]
E.García, Y.Diez, O.Diaz, X.Lladó, A.Gubern-Mérida, R.Martí, J.Martí, and A.Oliver.
Multimodal breast parenchymal patterns correlation using a patient-specific biomechanical model.
IEEE Trans. on Medical Imaging, 37(3), pp 712-723, 2018.
[IF 7.816, Q1(3/129) RNMMI]
- [NI-Cl 2018]
M.Salem, M.Cabezas, S.Valverde, D.Pareto, A.Oliver, J.Salvi, À.Rovira, and X.Lladó.
A supervised framework with intensity subtraction and deformation fields features for the detection of new T2-w lesions in multiple sclerosis.
NeuroImage: Clinical, 17, pp 607-615, 2018.
[IF 3.943, Q1(3/14) NI]
- [MP 2018]
E.García, Y.Diez, O.Diaz, X.Lladó, R.Martí, J.Martí, and A.Oliver.
A step-by-step review on patient-specific biomechanical finite elements models for breast MRI to X-ray mammography registration.
Medical Physics, 45(1), pp e6-e31, 2018.
[IF 3.177, Q2(32/129) RNMMI]
- [EJR 2017]
E.García, O.Diaz, R.Martí, Y.Diez, A.Gubern-Mérida, M.Sentís, J.Martí, and A.Oliver.
Local breast density assessment using reacquired mammographic images.
European Journal of Radiology, 93, pp 121-127, 2017.
[IF 2.843, Q2(38/128) RNMMI]
- [NI-Cl 2017]
S.González-Villà, S.Valverde, M.Cabezas, D.Pareto, J.C.Vilanova, Ll.Ramió-Torrentà, À.Rovira, A.Oliver, and X.Lladó.
Evaluating the effect of multiple sclerosis lesions on automatic brain structures segmentation.
Neuroimage: Clinical, 15, pp 228-238, 2017.
[IF 3.869, Q1(3/14) NI]
- [NI 2017]
S.Valverde, M.Cabezas, E.Roura, S.González-Villà, D.Pareto, J.C.Vilanova, Ll.Ramió-Torrentà, À.Rovira, A.Oliver, and X.Lladó.
Improving automated multiple sclerosis lesion segmentation with a cascaded 3D convolutional neural network approach.
Neuroimage, 155, pp 159-168, 2017.
[IF 5.426, Q1(1/14) NI]
- [MIA 2017]
S.Valverde, A.Oliver, E.Roura, S.González-Villà, D.Pareto, J.C.Vilanova, Ll.Ramió-Torrentà, À.Rovira, and X.Lladó.
Automated tissue segmentation of MR brain images in the presence of white matter lesions.
Medical Image Analysis, 35, pp 446-457, 2017.
[IF 5.356, Q1(10/132) CSAI]
- [IRBM 2017]
C.Mata, A.Oliver, A.Lalande, P.Walker, and J.Martí.
On the use of XML in medical imaging web-based applications.
IRBM, 38(1), pp 3-12, 2017.
[IF 0.897, Q4(69/78) EB]
- [AIIM 2016]
S.González-Villà, A.Oliver, S.Valverde, L.Wang, R.Zwiggelaar, and X.Lladó.
A review on brain structures segmentation in magnetic resonance imaging.
Artificial Intelligence in Medicine, 73, pp 45-69, 2016.
[IF 2.009, Q2(55/133) CSAI]
- [FNINF 2016]
E.Roura, N.Sarbu, A.Oliver, S.Valverde, S.González-Villà, R.Cervera, N.Bargalló, and X.Lladó.
Automated detection of lupus white matter lesions in MRI images.
Frontiers in Neuroinformatics, 10, art 33, 2016.
[IF 3.870, Q1(6/57) MCB]
- [AJNR 2016]
M.Cabezas, J.F.Corral, A.Oliver, Y.Diez, M.Tintoré, C.Auger, X.Montalban, X.Lladó, D.Pareto, and À.Rovira.
Improved automatic detection of new T2 lesions in multiple sclerosis using deformation fields.
American Journal of Neuroradiology, 37(10), pp 1816-1823, 2016.
[IF 3.550, Q1(22/127) RNMMI]
- [JMI 2016] J.Schwaab, Y.Diez, A.Oliver, R.Martí, J.van Zelst, A.Gubern-Mérida, A.Bensouda Mourri, J.Gregori, M.Günther. Automated quality assessment in 3D breast ultrasound images. Journal of Medical Imaging, 3(2), 027002, 2016.
- [IHSC 2016]
C.Mata, P.Walker, A.Oliver, F.Brunotte, J.Martí, and A.Lalande.
ProstateAnalyzer: web-based medical application for the management of prostate cancer using multiparametric MR images.
Informatics for Health & Social Care, 41(3), pp 286-306, 2016.
[IF 1.381, Q3(17/23) MI]
- [NI-Cl 2015]
S.Valverde, A.Oliver, E.Roura, D.Pareto, J.C.Vilanova, Ll.Ramió-Torrentà, J.Sastre-Garriga, X.Montalban, À.Rovira, and X.Lladó.
Quantifying brain tissue volume in multiple sclerosis with automated lesion segmentation and filling.
NeuroImage: Clinical, 9, pp 640-647, 2015.
[IF 3.857, Q1(3/14) NI]
- [NR 2015]
E.Roura, A.Oliver, M.Cabezas, S.Valverde, D.Pareto, J.C.Vilanova, Ll.Ramió-Torrentà, À.Rovira, and X.Lladó.
A toolbox for multiple sclerosis lesion segmentation.
Neuroradiology, 57(10), pp 1031-1043, 2015.
[IF 2.274, Q2(47/124) RNMMI]
- [JDI 2015]
A.Oliver, M.Tortajada, X.Lladó, J.Freixenet, S.Ganau, L.Tortajada,
M.Vilagran, M.Sentís, and R.Martí.
Breast density analysis using an automatic density segmentation algorithm.
Journal of Digital Imaging, 28(5), pp 604-612, 2015.
[IF 1.406, Q3(91/124) RNMMI]
- [AJNR 2015]
S.Valverde, A.Oliver, Y.Diez, M.Cabezas, J.C.Vilanova, Ll.Ramió-Torrentà, À.Rovira, and X.Lladó.
Evaluating the effects of white matter multiple sclerosis lesions on the volume estimation of six brain tissue segmentation methods.
American Journal of Neuroradiology, 36(6), pp 1109-1115, 2015.
[IF 3.124, Q1(24/124) RNMMI]
- [IJBC 2015] W.He, A.Juette, E.R.E.Denton, A.Oliver, R.Martí, and R.Zwiggelaar. A review on automatic mammography density and parenchymal segmentation. International Journal of Breast Cancer, ID 276217, 2015.
- [TBE 2015]
Z.Chen, H.Strange, A.Oliver, E.R.E.Denton, C.Boggis, and R.Zwiggelaar.
Topological modelling and classification of mammographic microcalcification clusters.
IEEE Transactions on Biomedical Engineering, 62(4), pp 1203-1214, 2015.
[IF 2.468, Q2(22/76) EB]
- [JMRI 2015]
S.Valverde, A.Oliver, M.Cabezas, E.Roura, and X.Lladó.
Comparison of ten brain tissue segmentation methods using revisited IBSR annotations.
Journal of Magnetic Resonance Imaging, 41(1), pp 93-101, 2015.
[IF 3.250, Q1(23/124) RNMMI]
- [JNM 2014]
M.Cabezas, A.Oliver, S.Valverde, B.Beltran, J.Freixenet, J.C.Vilanova, Ll.Ramió-Torrentà, À.Rovira, and X.Lladó.
BOOST: a supervised approach for multiple sclerosis lesion segmentation.
Journal of Neuroscience Methods, 237, pp 108-117, 2014.
[IF 2.025, Q3(174/252) N]
- [NI-Cl 2014]
S.Valverde, A.Oliver, X.Lladó.
A white matter lesion-filling approach to improve brain tissue volume measurements.
NeuroImage: Clinical, 6, pp 86-92, 2014.
[IF 2.526, Q2(6/14) NI]
- [NINF 2014]
Y.Diez, A.Oliver, M.Cabezas, S.Valverde, R.Martí, J.C.Vilanova, Ll.Ramió-Torrentà, À.Rovira, and X.Lladó.
Intensity based methods for brain MRI longitudinal registration.
A study on multiple sclerosis patients.
Neuroinformatics, 12(3), pp 365-379, 2014.
[IF 2.825, Q1(13/102) CSIA]
- [CBM 2014]
M.Tortajada, A.Oliver, R.Martí, S.Ganau, L.Tortajada, M.Sentís, J.Freixenet, and R.Zwiggelaar.
Breast peripheral area correction in digital mammograms.
Computers in Biology and Medicine, 50, pp 32-40, 2014.
[IF 1.240, Q3(64/102) CSIA]
- [CMPB 2014]
M.Cabezas, A.Oliver, E.Roura, J.Freixenet, J.C.Vilanova, Ll.Ramió-Torrentà, À.Rovira, and X.Lladó.
Automatic multiple sclerosis lesion detection in brain MRI by FLAIR thresholding.
Computer Methods and Programs in Biomedicine, 115(3), pp. 147-161, 2014.
[IF 1.897, Q1(15/102) CSTM]
- [NR 2014]
O.Ganiler, A.Oliver, Y.Díez, J.Freixenet, J.C.Vilanova, B.Beltrán, Ll.Ramió-Torrentà, À.Rovira, and X.Lladó.
A subtraction pipeline for automatic detection of new appearing multiple sclerosis lesions in longitudinal studies.
Neuroradiology, 56(5), pp 363-374, 2014 .
[IF 2.485, Q2(41/125) RNMMI]
- [CMPB 2014]
E.Roura, A.Oliver, M.Cabezas, J.C.Vilanova, Ll.Ramió-Torrentà, À.Rovira, and X.Lladó.
MARGA: Multispectral Adaptive Region Growing Algorithm for brain extraction on axial MRI.
Computer Methods and Programs in Biomedicine, 113(2), pp 655-673, 2014.
[IF 1.897, Q1(15/102) CSTM]
- [MIA 2013]
S.Ghose, A.Oliver, J.Mitra, R.Martí, X.Lladó, J.Freixenet, D.Sidibé, J.C.Vilanova, J.Comet, and F.Meriaudeau.
A supervised learning framework of statistical shape and probability priors for automatic prostate segmentation in ultrasound images.
Medical Image Analyis, 17(6), pp 587-600, 2013.
[IF 3.681, Q1(10/121) CSAI]
- [MIA 2012]
J.Mitra, Z.Kato, R.Martí, A.Oliver, X.Lladó, D.Sidibé, S.Ghose, J.C.Vilanova, J.Comet, and F.Meriaudeau.
A spline-based diffeomorphism for prostate multimodal registration.
Medical Image Analyis, 16(6), pp 1259-1279, 2012.
[IF 4.087, Q1(7/115) CSAI]
- [CMPB 2012]
S.Ghose, A.Oliver, R.Martí, X.Lladó, J.C.Vilanova, J.Freixenet, J.Mitra, D.Sidibé, and F.Meriaudeau.
A survey of prostate segmentation methodologies in ultrasound, magnetic resonance, and computed tomography images.
Computer Methods and Programs in Biomedicine, 108(1), pp 262-287, 2012.
[IF 1.555, Q1(21/100) CSTM]
- [NR 2012]
X.Lladó, O.Ganiler, A.Oliver, R.Martí, J.Freixenet, L.Valls, J.C.Vilanova, Ll.Ramió-Torrentà, and À.Rovira.
Automated detection of multiple sclerosis lesions in serial brain MRI.
Neuroradiology, 54(8), pp 787-807, 2012.
[IF 2.700, Q2(34/120) RNMMI]
- [IJCARS 2012]
J.Mitra, R.Martí, A.Oliver, X.Lladó, S.Ghose, J.C.Vilanova, and F.Meriaudeau.
Prostate multimodality image registration based on b-splines and quadrature local energy.
International Journal of Computer Assisted Radiology and Surgery, 7(3), pp 445-454, 2012.
[IF 1.364, Q3(76/120) RNMMI]
- [KBS 2012]
A.Oliver, A.Torrent, X.Lladó, M.Tortajada, L.Tortajada, M.Sentís, J.Freixenet, and R.Zwiggelaar.
Automatic microcalcification and cluster detection in digital and digitised mammograms.
Knowledge-Based Systems, 28, pp 68-75, 2012.
[IF 4.104, Q1(6/115) CSAI]
- [IS 2012]
X.Lladó, A.Oliver, M.Cabezas, J.Freixenet, J.C.Vilanova, A.Quiles, L.Valls, Ll.Ramió-Torrentà, and À.Rovira.
Segmentation of multiple sclerosis lesions in brain MRI: a review of automated approaches.
Information Sciences, 186(1), pp 164-185, 2012.
[IF 3.643, Q1(6/132) CSIS]
- [IJCARS 2012]
S.Ghose, A.Oliver, R.Martí, X.Lladó, J.Freixenet, J.Mitra, J.C.Vilanova, J.Comet, and F.Meriaudeau.
Statistical shape and texture model of quadrature phase information for prostate segmentation.
International Journal of Computer Assisted Radiology and Surgery, 7(1), pp 43-55, 2012.
[IF 1.364, Q3(76/120) RNMMI]
- [CMPB 2011]
M.Cabezas, A.Oliver, X.Lladó, J.Freixenet, and M.BachCuadra.
A review of atlas-based segmentation for magnetic resonance brain images.
Computer Methods and Programs in Biomedicine, 104(3), pp e158-e177, 2011.
[IF 1.516, Q1(14/99) CSTM]
- [T-ITB 2011]
Y.Díez, A.Oliver, X.Lladó, J.Freixenet, J.Martí, J.C.Vilanova, and R.Martí.
Revisiting intensity-based image registration applied to mammography.
IEEE Trans. on Information Technology in BioMedicine, 15(5), pp 716-725, 2011.
[IF 1.676, Q1(27/133) CSIS]
- [PRL 2011]
X.Lladó, A.DelBue, A.Oliver, J.Salvi, and L.Agapito.
Reconstruction of non-rigid 3D shapes from stereo-motion.
Pattern Recognition Letters, 32(7), pp 1020-1028, 2011.
[IF 1.034, Q3(63/111) CSAI]
- [AR 2010]
A.Oliver, X.Lladó, J.Freixenet, R.Martí, E.Pérez, J.Pont, and R.Zwiggelaar.
Influence of using manual or automatic breast density information in a mass detection CAD system.
Academic Radiology, 17(7), pp 877-883, 2010.
[IF 2.195, Q2(46/113) RNMMI]
- [MIA 2010]
A.Oliver, J.Freixenet, J.Martí, E.Pérez, J.Pont, E.R.E.Denton, and R.Zwiggelaar.
A review of automatic mass detection and segmentation in mammographic images.
Medical Image Analysis, 14(2), pp 87-110, 2010.
[IF 4.364, Q1(5/108) CSAI; Q1(1/97) CSIA]
- [IJEE 2010]
X.Lladó, J.Freixenet, and A.Oliver.
Linking research and teaching through an applied computer vision course.
International Journal of Engineering Education, 26(6), pp 1445-1454, 2010.
[IF 0.552, Q3(17/24) ESD]
- [JDI 2010]
A.Oliver, X.Lladó, E.Pérez, J.Pont, E.R.E.Denton, J.Freixenet, and J.Martí.
A statistical approach for breast density segmentation.
Journal of Digital Imaging, 23(5), pp 527-537, 2010.
[IF 1.421, Q3(68/113) RNMMI]
- [CMIG 2009]
X.Lladó, A.Oliver, J.Freixenet, R.Martí, and J.Martí.
A textural approach for mass false positive reduction in mammography.
Computerized Medical Imaging and Graphics, 33(6), pp 415-422, 2009.
[IF 1.041, Q4(79/104) RNMMI]
- [MP 2008]
J.Freixenet, A.Oliver, R.Martí, X.Lladó, J.Pont, E.Pérez, E.R.E.Denton, and R.Zwiggelaar.
Eigendetection of masses considering false positive reduction and breast density information.
Medical Physics, 35(5), pp 1840-1853, 2008.
[IF 3.871, Q1(13/92) RNMMI]
- [T-ITB 2008]
A.Oliver, J.Freixenet, R.Martí, J.Pont, E.Pérez, E.R.E.Denton, and R.Zwiggelaar.
A novel breast tissue density classification methodology.
IEEE Trans. on Information Technology in BioMedicine, 12(1), pp 55-65, 2008.
[IF 1.939, Q1(21/94) CSIA]
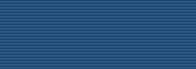
Abstracts in Medical Journals
- [MS 2024] A.Clèrigues, M.Guirao, R.Bramon, A.Tovar, M.A.Càmara, L.Valencia, R.Alcàntara, J.Jurado, R.Ocete, P.Pinero, F.Ramos, A.Oliver, X.Lladó, S.Valverde, and À.Rovira. Evaluating the performance of the AH-1 imaging software for the clinical management of MS patients in real world conditions. Congress of the European Committee for Treatment and Research in Multiple Sclerosis, Copenhagen (Denmark), September, 2024.
- [MS 2024] M.Guirao, S.Valverde, R.Bramon, A.Clèrigues, A.Tovar, M.A.Càmara, L.Valencia, N.Nerseyan, G.Álvarez, M.Puig, A.Gifreu, A.Oliver, X.Lladó, À.Rovira, and Ll.Ramió-Torrentà. Improving generalization of MRI-based detection of new T2-lesions through fine-tuning deep learning methods. Congress of the European Committee for Treatment and Research in Multiple Sclerosis, Copenhagen (Denmark), October, 2024.
- [MS 2023] Ll.Coll, P.Carbonell-Mirabent, Á.Cobo-Calvo, G.Arrambide, À.Vidal-Jordana, M.Comabella, J.Castilló, B.Rodríguez-Acevedo, A.Zabalza, I.Galan, L.Midaglia, C.Nos, A.Salerno, C.Auger, M.Alberich, J.Río, J.Sastre-Garriga, A.Oliver, X.Montalban, À.Rovira, M.Tintoré, D.Pareto, X.Lladó, and C.Tur. Prediction of progression independent of relapse activity at the first demyelinating attack with a deep learning image-based survival model. Congress of the European Committee for Treatment and Research in Multiple Sclerosis, Milano (Italy), October, 2023.
- [MS 2023] A.Clèrigues, S.Valverde, R.Bramon, L.Valencia, M.Guirao, J.Salvi, A.Oliver, À.Rovira, and X.Lladó. End to end multiple sclerosis lesions inpainting and tissue segmentation using a deep learning approach. Congress of the European Committee for Treatment and Research in Multiple Sclerosis, Milano (Italy), October, 2023.
- [MS 2023] A.Clèrigues, S.Valverde, R.Bramon, L.Valencia, M.Guirao, J.Salvi, A.Oliver, À.Rovira, and X.Lladó. Studying the effect of MS lesions on brain volumetry computed using a patch-based deep learning tissue segmentation. Congress of the European Committee for Treatment and Research in Multiple Sclerosis, Milano (Italy), October, 2023.
- [MS 2023] M.Guirao, N.Nerseyan, S.Valverde, R.Bramon, A.Clèrigues, L.Valencia, G.Álvarez, M.Puig, A.Gifreu, A.Oliver, X.Lladó, À.Rovira, and Ll.Ramió-Torrentà. Inclusion of attention gates in a 3D CNN model improves the performance in new T2 lesion quantification. Congress of the European Committee for Treatment and Research in Multiple Sclerosis, Milano (Italy), October, 2023.
- [MS 2022]
S.Valverde, R.Bramon, A.Clèrigues, L.Valencia, A.Oliver, N.Nerseyan, M.Puig, R.Robles, G.Álvarez, D.Lourido, Ll.Ramió-Torrentà, À.Rovira, and X.Lladó.
Assessing the performance of an automated decision-support system for detecting active T2 lesions on non-standardized MRI systems and field strengths.
Multiple Sclerosis, 28(S3), pp 271,
2022.
[IF 5.885, Q1(40/212) CN]
- [MS 2022]
À.Rovira, S.Sceppacuercia, S.Valverde, C.Auger, J.F.Corral, A.Vidal-Jordana, R.Bramon, A.Oliver, M.Tintoré, D.Pareto, F.X.Aymerich, X.Montalban, X.Lladó, and J.Alonso.
Evaluation of a supervised approach based on the application of CNN for the recognition of new T2 lesions in patients with multiple sclerosis using different MR magnets..
Multiple Sclerosis, 27(S2), pp 290-291,
2022.
[IF 5.885, Q1(40/212) CN]
- [MS 2022]
Ll.Coll, P.Carbonell-Mirabent, Á.Cobo-Calvo, G.Arrambide, À.Vidal-Jordana, M.Comabella, J.Castilló-Justribó, B.Rodríguez-Acevedo, A.Zabalza, I.Galan, L.Midaglia, C.Nos, A.Salerno, C.Auger, M.Alberich, J.Río, J.Sastre-Garriga, A.Oliver, X.Montalban, À.Rovira, M.Tintoré, D.Pareto, X.Lladó, and C.Tur.
Convolutional neural networks on routinely-acquired MRI to stratify MS patients according to their disability level and understand anatomical regions involved in clinical progression.
Multiple Sclerosis, 28(S3), pp 18-19,
2022.
[IF 5.885, Q1(40/212) CN]
- [MS 2021]
L.Valencia, S.Valverde, A.Clèrigues, A.Oliver, J.F.Corral, À.Rovira, and X.Lladó.
Assessing the usability of synthetic images to improve the detection of new T2 lesions.
Multiple Sclerosis, 27(S2), pp 480,
2021.
[IF 5.885, Q1(40/212) CN]
- [MS 2020]
J.F.Corral, J.Alonso, C.Auger, S.Valverde, A.Vidal-Jordana, A.Oliver, A.Barros, K.Ng Wong, M.Tintoré, F.X.Aymerich, X.Montalban, X.Lladó, and À.Rovira.
Assessment of automatic decision-support systems for detecting active T2 lesions in multiple sclerosis patients.
Multiple Sclerosis, 26(S3), pp 375-376,
2020.
[IF 5.412, Q1(26/204) CN]
- [MS 2019]
S.Gonzàlez-Villá, S.Valverde, M.Cabezas, Y.Huo, A.Oliver, Ll.Ramió-Torrentà, B.A.Landman, and X.Lladó.
An end to end automated pipeline for brain structure segmentation in multiple sclerosis patients.
Multiple Sclerosis, 25(S2), pp 256-257,
2019.
[IF 5.412, Q1(26/204) CN]
- [MS 2019]
M.Salem, S.Valverde, M.Cabezas, D.Pareto, A.Oliver, J.Salvi, À.Rovira, and X.Lladó.
Detecting the appearance of new T2-w multiple sclerosis lesions in longitudinal studies using deep convolutional neural networks.
Multiple Sclerosis, 25(S2), pp 462-463,
2019.
[IF 5.412, Q1(26/204) CN]
- [MS 2019]
M.Salem, S.Valverde, M.Cabezas, D.Pareto, A.Oliver, J.Salvi, À.Rovira, and X.Lladó.
Lesion synthesis for extending MRI training datasets and improving automatic multiple sclerosis lesion segmentation.
Multiple Sclerosis, 25(S2), pp 463,
2019.
[IF 5.412, Q1(26/204) CN]
- [MS 2018]
S.Valverde, M.Salem, M.Cabezas, D.Pareto, J.C.Vilanova, Ll.Ramió-Torrentà, À.Rovira, A.Oliver, J.Salvi, and X.Lladó.
Manual delineation of only one image in unseen databases is sufficient for accurate performance in automated multiple sclerosis lesion segmentation.
Multiple Sclerosis, 24(S2), pp 222,
2018.
[IF 5.649, Q1(22/197) CN]
- [MS 2018]
S.Valverde, M.Salem, M.Cabezas, D.Pareto, J.C.Vilanova, Ll.Ramió-Torrentà, À.Rovira, A.Oliver, J.Salvi, and X.Lladó.
Manual delineation of only one image in unseen databases is sufficient for accurate performance in automated multiple sclerosis lesion segmentation.
Multiple Sclerosis, 24(S2), pp 222,
2018.
[IF 5.649, Q1(22/197) CN]
- [MS 2018]
A.Clèrigues, S.Valverde, J.Bernal, D.Pareto, J.C.Vilanova, Ll.Ramió-Torrentà, À.Rovira, A.Oliver, and X.Lladó.
A quantitative analysis of deep learning methods for multiple sclerosis white matter lesion segmentation.
Multiple Sclerosis, 24(S2), pp 637-638,
2018.
[IF 5.649, Q1(22/197) CN]
- [MS 2017]
S.Valverde, M.Cabezas, E.Roura, S.González-Villà, D.Pareto, J.C.Vilanova, Ll.Ramió-Torrentà, À.Rovira, A.Oliver, and X.Lladó.
A deep learning approach for multiple sclerosis lesion segmentation.
Multiple Sclerosis, 23(S3), pp 531-532,
2017.
[IF 5.280, Q1(22/197) CN]
- [MS 2017]
S.González-Villà, S.Valverde, M.Cabezas, D.Pareto, J.C.Vilanova, Ll.Ramió-Torrentà, À.Rovira, A.Oliver, and X.Lladó.
Do multiple sclerosis lesions affect automatic brain structure segmentation.
Multiple Sclerosis, 23(S3), pp 257-258,
2017.
[IF 5.280, Q1(22/197) CN]
- [MS 2017]
M.Salem, M.Cabezas, S.Valverde, D.Pareto, A.Oliver, J.Salvi, À.Rovira, and X.Lladó.
Supervised detection of newly appearing T2-w multiple sclerosis lesions with subtraction and deformation fields features.
Multiple Sclerosis, 23(S3), pp 794,
2017.
[IF 5.280, Q1(22/197) CN]
- [MS 2016]
S.Valverde, A.Oliver, S.González-Villà, E.Roura, D.Pareto, J.C.Vilanova, Ll.Ramió-Torrentà, À.Rovira, and X.Lladó.
Automated tissue segmentation of magnetic resonance images of multiple sclerosis patients.
Multiple Sclerosis, 22(S3), pp 757,
2016.
[IF 4.840, Q1(27/192) CN]
- [MS 2016]
M.Cabezas, D.Pareto, A.Oliver, J.F.Corral, C.Auger, X.Aymerich, J.Sastre-Garriga, M.Tintoré, X.Montalban, X.Lladó, and À.Rovira.
Detection of new multiple sclerosis lesions on longitudinal brain MRI.
Multiple Sclerosis, 22(S3), pp 215-216,
2016.
[IF 4.840, Q1(27/192) CN]
- [MS 2015]
S.Valverde, A.Oliver, E.Roura, D.Pareto, J.C.Vilanova, Ll.Ramió-Torrentà, J.Sastre-Garriga, X.Montalban, À.Rovira, and X.Lladó.
Evaluation of two automated lesion segmentation and filling pipelines for brain tissue segmentation of multiple sclerosis patients.
Multiple Sclerosis, 23(S11), pp 177-178,
2015.
[IF 4.671, Q1(27/192) CN]
- [MS 2015]
E.Roura, A.Oliver, M.Cabezas, S.Valverde, D.Pareto, J.C.Vilanova, Ll.Ramió-Torrentà, À.Rovira, and X.Lladó.
A toolbox for segmenting multiple sclerosis lesions using T1w and FLAIR images.
Multiple Sclerosis, 23(S11), pp 678,
2015.
[IF 4.671, Q1(27/192) CN]
- [MS 2015]
M.Cabezas, A.Oliver, X.Lladó, C.Auger, J.Sastre-Garriga, D.Pareto, X.Montalban, and À.Rovira.
A pipeline for detecting new multiple sclerosis lesions on longitudinal brain magnetic resonance imaging.
Multiple Sclerosis, 23(S11), pp 179-180,
2015.
[IF 4.671, Q1(27/192) CN]
- [MS 2015]
M.Cabezas, J.F.Corral, A.Oliver, X.Lladó, C.Auger, J.Sastre-Garriga, M.Tintoré, D.Pareto, X.Montalban, and À.Rovira.
Evaluating the sensitivity of subtraction imaging for the detection of new T2 multiple sclerosis lesions when using visual analysis.
Multiple Sclerosis, 23(S11), pp 175,
2015.
[IF 4.671, Q1(27/192) CN]
- [MS 2014]
S.Valverde, A.Oliver, D.Pareto, J.C.Vilanova, À.Rovira, Ll.Ramió-Torrentà, and X.Lladó.
SLF: a MS white matter lesion filling toolbox for the SPM software.
Multiple Sclerosis, 20(S1), pp 296,
2014.
[IF 4.822, Q1(22/192) CN]
- [MS 2013]
E.Roura, A.Oliver, M.Cabezas, O.Ganiler, J.Freixenet, X.Lladó, J.C.Vilanova, À.Rovira, and Ll.Ramió-Torrentà.
Extracting the brain in axial MRI images with and adaptive segmentation technique.
Multiple Sclerosis, 19(S1), pp 417-418,
2013.
[IF 4.863, Q1(23/194) CN]
- [MS 2013]
S.Valverde, X.Lladó, M.Cabezas, Y.Díez, J.Freixenet, A.Oliver, J.C.Vilanova, À.Rovira, and Ll.Ramió-Torrentà.
A quantitative study of the effects of white matter multiple sclerosis lesions on tissue segmentation methods.
Multiple Sclerosis, 19(S1), pp 407-408,
2013.
[IF 4.863, Q1(23/194) CN]
- [NE 2012]
X.Lladó, M.Cabezas, O.Ganiler, A.Oliver, Y.Donoso, J.Freixenet, L.Valls, A.Quiles, G.Laguillo, D.Pareto, J.C.Vilanova, À.Rovira, and Ll.Ramió-Torrentà.
SALEM: herramientas informáticas para la detección de lesiones de esclerosis
múltiple en estudios longitudinales mediante imágenes
de resonancia magnética del cerebro.
Neurología, pp 195-196,
2012,
[IF 1.322, Q3(143/191) CN]
- [MS 2012]
M.Cabezas, A.Oliver, X.Lladó, Y.Díez, J.Freixenet, J.C.Vilanova, A.Quiles, G.Laguillo, Ll.Ramió-Torrentà, D.Pareto, and À.Rovira.
A supervised approach to segment multiple sclerosis lesions using context-rich features and a boosting classifier.
Multiple Sclerosis, 18(S4), pp 157,
2012.
[IF 4.472, Q1(26/193) CN]
- [MS 2012]
O.Ganiler, X.Lladó, A.Oliver, Y.Díez, J.Freixenet, J.C.Vilanova, A.Quiles, G.Laguillo, Ll.Ramió-Torrentà, D.Pareto, and À.Rovira.
Detecting evolving white matter MS lesions in serial brain MRI studies: analysis of a subtraction approach.
Multiple Sclerosis, 18(S4), pp 385,
2012.
[IF 4.472, Q1(26/193) CN]
- [MS 2012]
Y.Díez, X.Lladó, A.Oliver, R.Martí, E.Roura, M.Cabezas, O.Ganiler, J.Freixenet, J.C.Vilanova, L.Valls, Ll.Ramió-Torrentà, D.Pareto, and À.Rovira.
Registration of serial brain MRI scans from multiple sclerosis patients. Analysis of 3D intensity-based methods.
Multiple Sclerosis, 18(S4), pp 384-385,
2012.
[IF 4.472, Q1(26/193) CN]
- [NE 2011]
J.Freixenet, M.Cabezas, M.BachCuadra, A.Oliver, X.Lladó, J.C.Vilanova, L.Valls, Ll.Ramió-Torrentà, E.Huerga, D.Pareto, and À.Rovira.
Segmentación de lesiones de esclerosis múltiple en resonancia magnética del cerebro mediante información espacial.
Neurología, 26(Sup), pp 182, 2011
[IF 0.790, Q4(161/191) CN]
- [NE 2011]
X.Lladó, O.Ganiler, M.Cabezas, A.Oliver, J.Freixenet, J.C.Vilanova, L.Valls, Ll.Ramió-Torrentà, and À.Rovira.
Técnicas automàticas de segmentaciòn de lesiones de EM y de cuantificación volumètrica en estudios temporales.
Neurología, 26(Sup), pp 181-182, 2011
[IF 0.790, Q4(161/191) CN]
- [MS 2011]
M.Cabezas, M.BachCuadra, A.Oliver, X.Lladó, J.Freixenet, J.C.Vilanova, L.Valls, Ll.Ramió-Torrentà, E.Huerga, D.Pareto, and À.Rovira.
A pipeline approach with spatial information for segmenting multiple sclerosis lesions on brain magnetic resonance imaging.
Multiple Sclerosis, 17(10S), pp 381, 2011.
[IF 4.255, Q1(27/191) CN]
- [MS 2011]
X.Lladó, O.Ganiler, A.Oliver, M.Cabezas, J.Freixenet, J.C.Vilanova, L.Valls, Ll.Ramió-Torrentà, and À.Rovira.
Computer-assisted strategies to automated quantification of multiple sclerosis lesion evolution on brain magnetic resonance imaging.
Multiple Sclerosis, 17(10S), pp 161-162, 2011.
[IF 4.255, Q1(27/191) CN]
- [IJCARS 2011]
J.Mitra, F.Meriaudeau, R.Martí, A.Oliver, X.Lladó, S.Ghose, and J.C.Vilanova.
Quadrature filter enhanced b-spline registration applied to prostate multimodal images.
International Journal of Computer Assisted Radiology and Surgery, 6(s1), pp 323-324, 2011.
[IF 1.481, Q2(89/198) S]
- [IJCARS 2011]
S.Ghose, A.Oliver, R.Martí, X.Lladó, J.Freixenet, J.C.Vilanova, F.Meriaudeau, and J.Mitra.
Quadrature phase-based statistical shape and appearance for prostate segmentation.
International Journal of Computer Assisted Radiology and Surgery, 6(s1), pp 12-13, 2011.
[IF 1.481, Q2(89/198) S]
- [MS 2010]
X.Lladó, M.Cabezas, O.Ganiler, A.Oliver, J.Freixenet, J.C.Vilanova, A.Quiles, Ll.Ramió-Torrentà and À.Rovira.
Strategies for automated segmentation of multiple sclerosis lesions on brain magnetic resonance imaging .
Multiple Sclerosis, 16(10S), pp 256, 2010.
[IF 4.230, Q1(29/185) CN]
- [IJCARS 2010] A.Oliver, X.Lladó, J.Freixenet, and J.Martí. Applying Zernike moments for automatic mass diagnosis. International Journal of Computer Assisted Radiology and Surgery, 5(s1), pp 201-202, 2010.
- [IJCARS 2008] A.Oliver, J.Freixenet, X.Lladó, R.Martí, J.Pont, E.Pérez, and R.Zwiggelaar. Comparing the performance of a mass detection CAD system when using manual and automatic breast density information. International Journal of Computer Assisted Radiology and Surgery, 3(s1), pp 420-421, 2008.
- [IJCARS 2008] R.Martí, J.Martí, J.Freixenet, J.C.Vilanova, J.Barceló, A.Gubern, A.Oliver, and X.Lladó. Intensity based MRI/TRUS data fusion for prostatic guided biopsy. International Journal of Computer Assisted Radiology and Surgery, 3(s1), pp 369-370, 2008.
- [IJCARS 2007] J.Freixenet, A.Oliver, X.Lladó, R.Martí, and J.Martí. Mass Eigendetection and the Benefits of Introducing Breast Density Information International Journal of Computer Assisted Radiology and Surgery, 2(s1), pp 332-334, 2007.
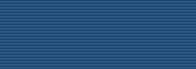
My PhD Thesis
- [PhD Arnau Oliver 2007] A.Oliver. Automatic Mass Segmentation in Mammographic Images. European Thesis (supervisor: Dr. Jordi Freixenet). University of Girona, Spain. July 2007.
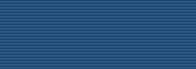
Books, Book Chapters & Editor
- [ETC2 2022] A.Oliver, X.Lladó, P.Vilà, J.Freixenet, and X.Cufí. Estructura i tecnologia de computadors. Màquina senzilla: Teoria i exercicis. Documenta Universitaria, ISBN: 978-8499846279. 2022.
- [WS 2022] J.Bernal, K.Kushibar, A.Clèrigues, A.Oliver, and X.Lladó. Deep learning from medical imaging. Deep learning in biology and medicine, chapter 2. Eds: D.Bacciu, P.J.G.Lisboa, A.Vellido, World Scientific. 2022.
- [ETC 2015] A.Oliver, J.Salvi, A.Martorano, J.Batlle. Estructura i tecnologia de computadors. Documenta Universitaria, ISBN: 978-8499842981. 2015.
- [ETC2 2014] A.Oliver, X.Lladó, X.Cufí, J.Ferrer, M.Carreras, and M.Fàbregas. Estructura i tecnologia de computadors: MIPS 32. Documenta Universitaria, ISBN: 978-8499842313. 2014.
- [BSP 2011] J.Martí, A.Gubern-Mérida, J.Massich, A.Oliver, J.C.Vilanova, J.Comet, E.Pérez, M.Arzoz, and R.Martí. Ultrasound Image Analysis. Methods and Applications. Recent Advances in Biomedical Signal Processing, pp 216-230. Eds: J.M.Górriz, E.W.Lang, and J.Ramírez, Bentham Science Publishers. 2011.
- [IWDM 2010] J.Martí, A.Oliver, J.Freixenet, R.Martí. Digital Mammography, Proceedings of the 10th International Workshop. Lecture Notes in Computer Science, vol 6136. 2010.
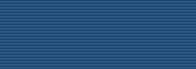
Participation in Challenges
- [MICCAI-TOPCOW 2024] R.Hamadache, C.Lisazo, C.Yalçin, V.Abramova, A.Casamitjana, A.Oliver, and X.Lladó. Segmentation of the Circle of Willis for CTA and MRA images. MICCAI - Topology-aware anatomical segmentation of the Circle of Willis Challenge. Marrakesh, Morocco. October 2024.
- [MICCAI-BRATS 2024] V.Abramova, U.M.Lal-Trehan, C.Yalçin, R.Hamadache, C.Lisazo, A.Casamitjana, J.Salvi, A.Oliver, and X.Lladó. nnUnet for meningioma segmentation in radiotherapy planning MRI. MICCAI - BraTS challenge on meningioma segmentation. Marrakesh, Morocco. October 2024.
- [MICCAI-MBH 2024] C.Yalçin, V.Abramova, R.Hamadache, U.M.Lal-Trehan, C.Lisazo, A.Casamitjana, A.Oliver, and X.Lladó. nnUnet-based semi-supervise multi-class brain hemorrhage segmentation in non-contrast CT with 3D cross pseudo supervision. MICCAI - Multi-class brain hemorrhage segmentatino in non-contrast CT. Marrakesh, Morocco. October 2024.
- [MICCAI-FETA 2024] R.Hamadache, A.Bouzid, R.Montoya, M.Elbatel, A.Casamitjana, A.Oliver, R.Martí and X.Lladó. Segmentation of the FETA. MICCAI - Fetal tissue annotation and segmentation challenge. Marrakesh, Morocco. October 2024.
- [MICCAI-TOPCOW 2023] C.Yalçin, R.Hamadache, J.Salvi, A.Oliver, and X.Lladó. Segmentation of the Circle of Willis for CTA and MRA images using nnUNet. MICCAI - Topology-aware anatomical segmentation of the Circle of Willis Challenge. Vancouver, Canada. October 2023.
- [MICCAI-TOPCOW 2023] U.Lal-Trehan, V.Abramova, L.Giancardo, A.Oliver, and X.Lladó. Topology-aware anatomical segmentation of the Circle of Willis for CTA and MRA. MICCAI - Topology-aware anatomical segmentation of the Circle of Willis Challenge. Vancouver, Canada. October 2023.
- [MICCAI-BRATS 2023] V.Abramova, U.Lal-Trehan, C.Yalçin, R.Hamadache, A.Clèrigues, A.Tovar, M.Guirao, J.Salvi, A.Oliver, and X.Lladó. nnUnet for brain tumor segmentation in sub-saharian Africa patient population. MICCAI - BraTS challenge on Sub-Sahara-Africa adult glioma. Vancouver, Canada. October 2023.
- [MICCAI-ISLES 2022] A.Clèrigues, V.Abramova, L.Valencia, U.Lal-Trehan, M.Guirao, J.Salvi, A.Oliver, and X.Lladó. Stroke lesion segmentation from multimodal MRI using a residual U-net and bilateral modality augmentation. MICCAI - Ischemic stroke lesion segmentation in medical imaging. Singapur. September 2022.
- [MICCAI-INSTANCE 2022] V.Abramova, A.Clèrigues, L.Valencia, U.Lal-Trehan, M.Guirao, A.Oliver, J.Salvi, and X.Lladó. Intracranial hemorrhage segmentation using a 3D U-net with squeeze-and-excitation blocks. MICCAI - Intracranial hemorrhage segmentation challenge on non-contrast head CT. Singapur. September 2022.
- [MICCAI-CSeg 2022] E.E. Carvajal-Camelo, J.Bernal, A.Oliver, and X.Lladó. Infant cerebellum MRI segmentation using U-net. MICCAI - Grand challenge on multi-domain cross-time-point infant cerebellum MRI segmentation 2022. Singapur. September 2022.
- [MICCAI-MSSeg 2021] M.Salem, A.Oliver, J.Salvi, and X.Lladó. A fully convolutional neural network for the detection of new T2-w lesion in multiple sclerosis. MICCAI - Multiple sclerosis lesions segmentation challenge using a data management and processing infrastructure. Online. September 2021.
- [MICCAI-MSSeg 2021] M.Salem, A.Oliver, J.Salvi, and X.Lladó. A fully convolutional neural network for the detection of new T2-w lesion in multiple sclerosis. MICCAI - Multiple sclerosis lesions segmentation challenge using a data management and processing infrastructure. Online. September 2021.
- [MICCAI-iSeg 2019] J.Bernal, K.Kushibar, S.Valverde, M.Cabezas, M.Salem, J.Salvi, A.Oliver, and X.Lladó. Six-month infant brain magnetic resonance image tissue segmentation using multi-atlas segmentation with joint label fusion and convolutional neural networks. MICCAI - 6-month Infant Brain MRI Segmentation Grand Challenge. Shenzhen, China. October 2019.
- [MICCAI-BraTS 2019] M.Cabezas, S.Valverde, A.Clèrigues, M.Salem, K.Kushibar, J.Bernal, A.Oliver, J.Salvi, and X.Lladó. Brain tumour segmentation and prediction via CNNs. MICCAI - Multimodal Brain Tumor Segmentation Challenge. Shenzhen, China. October 2019.
- [MICCAI-ISLES 2018] A.Clèrigues, S.Valverde, J.Bernal, K.Kushibar, M.Cabezas, A.Oliver, and X.Lladó. Ensemble of convolution neural networks for acute stroke anatomy differentiation. MICCAI - Ischemic Stroke Lesion Segmentation Challenge. Granada, Spain. September 2018.
- [MICCAI-MRBrainS 2018] J.Bernal, M.Salem, K.Kushibar, A.Clèrigues, S.Valverde, M.Cabezas, S.González-Villà, J.Salvi, A.Oliver, and X.Lladó. MR brain segmentation using an ensemble of multi-path u-shaped convolutional neural networks and tissue segmentation priors. MICCAI - Grand Challenge on MR Brain Segmentation. Granada, Spain. September 2018.
- [MICCAI-BraTS 2018] M.Cabezas, S.Valverde, S.González-Villà, A.Clèrigues, M.Salem, K.Kushibar, J.Bernal, A.Oliver, J.Salvi, and X.Lladó. Survival prediction using ensemble tumor segmentation and transfer learning. MICCAI - Multimodal Brain Tumor Segmentation Challenge. Granada, Spain. September 2018.
- [MICCAI-iSeg 2017] J.Bernal, K.Kushibar, S.Valverde, M.Cabezas, S.González-Villà, M.Salem, J.Salvi, A.Oliver, and X.Lladó. Six-month infant brain tissue segmentation using three dimensional fully convolution neural networks and pseudo-labelling. MICCAI - 6-month Infant Brain MRI Segmentation Grand Challenge. Quebec, Canada. October 2017.
- [MICCAI-WMH 2017] S.Valverde, M.Cabezas, J.Bernal, K.Kushibar, S.González-Villà, M.Salem, J.Salvi, A.Oliver, and X.Lladó. White matter hyperintensities segmentation using a cascade of three convolutional neural networks. MICCAI - White Matter Hyperintensities Segmentation Grand Challenge. Quebec, Canada. October 2017.
- [MICCAI-MSSEG 2016] E.Roura, M.Cabezas, S.Valverde, S.González-Villà, J.Salvi, A.Oliver, and X.Lladó. Unsupervised multiple sclerosis lesion detection and segmentation using rules and level sets. MICCAI - Multiple Sclerosis Lesion Segmentation Grand Challenge. Athens, Greece. October 2016.
- [MICCAI-MSSEG 2016] S.Valverde, M.Cabezas, E.Roura, S.González-Villà, J.Salvi, A.Oliver, and X.Lladó. Multiple sclerosis lesion detection and segmentation using a convolutional neural network of 3D patches. MICCAI - Multiple Sclerosis Lesion Segmentation Grand Challenge. Athens, Greece. October 2016.
- [MICCAI-PROMISE 2012] S.Ghose, J.Mitra, A.Oliver, R.Martí, X.Lladó, J.Freixenet, J.C.Vilanova, D.Sidibé, and F.Meriaudeau. A random forest based classification approach to prostate segmentation in MRI. MICCAI Grand Challenge: Prostate MR image segmentation, pp 20-27. Nice, France. October 2012.
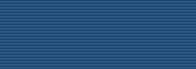
Papers in Conferences
- [AIH 2024] U.Lal-Trehan, S.Sheth, A.Oliver, X.Lladó, and L.Giancardo. Learnable 3D pooling for 3D-to-2D transformation of brain CT-Angiography. AI in Health Conference, Proc AIH, to appear. Houston, Texas. September 2024.
- [ACM-BCB 2023] U.Lal-Trehan, A.Oliver, S.Sheth, X.Lladó, and L.Giancardo. Strategies to combine 3D vasculature and brain CTA with deep neural networks: application to LVO. ACM Conference on Bioinformatics, Computational Biology, and Health Informatics, Proc ACM-BCB, to appear. Houston, Texas. September 2023.
- [IWDM 2022] E.García, R.Martí, J.Martí, J.del Riego, C.Aynes, A.Oliver, and O.Diaz. Simultaneous pectoral muscle and nipple location in MLO mammograms considering image quality assumptions. International Workshop on Breast Imaging, Proc. SPIE 12286, pp 122860E. Leuven, Belgium. May 2022.
- [MICCAI-MLCN 2020] S.Tascon-Morales, S.Hoffmann, M.Treiber, D.Mensing, A.Oliver, M.Guenther, and J.Gregori. Multiple sclerosis lesion segmentation using longitudinal normalization and convolutional recurrent neural networks. MICCAI - International Workshop on Machine Learning in Clinical Neuroimaging, LNCS 12449, pp 148-158. Lima, Peru (Virtual). October 2020.
- [IWDM 2020] E.García, Y.Diez, A.Oliver, N.Karssemeijer, J.Martí, R.Martí, and O.Diaz. Evaluation of elastic parameters for breast compression using a MRI-mammography registration approach. International Workshop on Breast Imaging, Proc. SPIE 11513, pp 1151306. Leuven, Belgium. May 2020.
- [MICCAI-PATCH 2018] S.González-Villà, Y.Huo, A.Oliver, X.Lladó, and B.Landman. Multi-atlas parcellation in the presence of lesions: application to multiple sclerosis. MICCAI - International Worshop on Patch-based Techniques in Medical Imaging, LNCS 11075, pp 104-113. Granada, Spain. September 2018.
- [MIUA 2018] N.Alam, A.Oliver, E.Denton, and R.Zwiggelaar. Automatic segmentation of microcalcifcation clusters. Medical Image Understanding and Analysis, pp 251-261. Southampton, UK. July 2018.
- [IWDM 2018] E.García, A.Oliver, O.Diaz, Y.Diez, A.Gubern-Mérida, J.Martí, and R.Martí. Changes in breast density over time using automatic density measures. Preliminary analysis. International Workshop on Breast Imaging, Proc. SPIE 10718, pp 107181l. Atlanta, Georgia. June 2018.
- [IbPRIA 2017] E.García, A.Oliver, Y.Diez, O.Diaz, X.Lladó, R.Martí, and J.Martí. Similarity metrics for intensity-based registration using breast density maps. Iberian Conference on Pattern Recognition and Image Analysis, to appear. Faro, Portugal. June 2017.
- [SPIE 2017] E.García, A.Oliver, O.Diaz, Y.Diez, R.Martí, and J.Martí. Mapping 3D breast lesions from full-field digital mammograms using subject-specific finite element models. SPIE Conference on Medical Imaging, Proc. SPIE 10135, pp 1013504O1-1013504O8. Orlando, Florida. February 2017.
- [SPIE 2017] O.Diaz, E.García, A.Oliver, J.Martí, and R.Martí. Scattered radiation in DBT geometries with flexible breast compression paddles: a monte carlo simulation study. SPIE Conference on Medical Imaging, Proc. SPIE 10132, pp 101324G1-101324G7. Orlando, Florida. February 2017.
- [IWDM 2016] E.García, A.Oliver, Y.Diez, O.Diaz, A.Gubern-Mérida, X.Lladó, and J.Martí. Comparison of four breast tissue segmentation algorithms for multi-modal MRI to X-ray mammography registration. International Workshop on Breast Imaging, LNCS 9699, pp 493-500. Malmö, Sweden. June 2016.
- [IWDM 2016] O.Diaz, A.Oliver, S.Ganau, J.Martí, M.Sentís, and R.Martí. Feasibility of depth sensors to study breast deformation during mammography procedures. International Workshop on Breast Imaging, LNCS 9699, pp 446-453. Malmö, Sweden. June 2016.
- [SPIE 2016] E.Roura, A.Oliver, M.Cabezas, S.Valverde, D.Pareto, J.C.Vilanova, Ll.Ramió-Torrentà, À.Rovira, and X.Lladó. An SPM12 extension for multiple sclerosis lesion segmentation. SPIE Conference on Medical Imaging, Proc SPIE 9784, pp 97842N1-97842N6. San Diego, California. February 2016.
- [MICCAI-BIA 2015] E.García, A.Oliver, Y.Diez, O.Diaz, J.Georgii, A.Gubern-Mérida, R.Martí, and J.Martí. Comparing regional breast density using full-field digital mammograms and magnetic resonance imaging: a preliminary study. MICCAI Workshop on Breast Image Analysis, pp 33-40. Munich, Germany. October 2015.
- [ICIP 2014] A.Oliver, X.Lladó, A.Torrent, and J.Martí. One-shot segmentation of breast, pectoral muscle, and background in digitised mammograms. IEEE International Conference on Image Processing, pp 912-916. Paris, France. October 2014.
- [IWDM 2014] R.Martí, Y.Diez, A.Oliver, M.Tortajada, R.Zwiggelaar, and X.Lladó. Detecting abnormal mammographic cases in temporal studies using image registration features. International Workshop on Breast Imaging, LNCS 8539, pp 612-619. Gifu, Japan. July 2014.
- [IWDM 2014] J.Massich, F.Meriaudeau, M.Sentís, S.Ganau, E.Pérez, D.Puig, R.Martí, A.Oliver, and J.Martí. SIFT texture description for understanding breast ultrasound images. International Workshop on Breast Imaging, LNCS 8539, pp 681-688. Gifu, Japan. July 2014.
- [BP 2013] A.Martorano, A.Oliver, J.Batlle, and J.Salvi. Prova pilot: l'assignatura Estructura i Tecnologia de Computadors (grau d'Enginyeria Informàtica) amb auto aprenentatge en format digital utilitzant problemes. Jornades de Bones Pràctiques, pp 19-25. Girona, Spain. September 2013.
- [BP 2013] X.Lladó and A.Oliver. Informàtica dels Sistems de Producció: aplicació de diferents tècniques d'aprenentatge en un grup reduït d'alumnes. Jornades de Bones Pràctiques, pp 70-73. Girona, Spain. September 2013.
- [WSRFAI 2013] C.Mata, A.Oliver, and J.Martí. A web-based tool for training radiologists in medical imaging. Workshop de Reconocimiento de Formas y Análisis de Imágenes, pp 5-8. Madrid, Spain. September 2013.
- [IbPRIA 2013] M.Cabezas, A.Oliver, J.Freixenet, and X.Lladó. A supervised approach for multiple sclerosis lesion segmentation using context features and an outlier map. Iberian Conference on Pattern Recognition and Image Analysis, LNCS 7887, pp 782-789. Madeira, Portugal. June 2013.
- [IbPRIA 2013] Z.Chen, A.Oliver, E.R.E.Denton, and R.Zwiggelaar. Automated mammographic risk classification based on breast density estimation. Iberian Conference on Pattern Recognition and Image Analysis, LNCS 7887, pp 237-244. Madeira, Portugal. June 2013.
- [BIBE 2012] C.Mata, A.Oliver, A.Torrent, and J.Martí. MammoApplet: an interactive Java applet tool for manual annotation in medical imaging. International Conference on Bioinformatics & Bioengineering, pp 34-39. Larnaca, Cyprus. November 2012.
- [ICPR 2012] S.Ghose, J.Mitra, A.Oliver, R.Martí, X.Lladó, J.Freixenet, J.C.Vilanova, D.Sidibé, and F.Meriaudeau. A Mumford-Shah functional based variational model with contour, shape and probability prior information for prostate segmentation. International Conference on Pattern Recognition, pp 121-124. Tsukuba Science City, Japan. November 2012.
- [ICPR 2012] S.Ghose, J.Mitra, A.Oliver, R.Martí, X.Lladó, J.Freixenet, J.C.Vilanova, D.Sidibé, and F.Meriaudeau. Graph cut energy minimization in a probabilistic learning framework for 3D prostate segmentation in MRI. International Conference on Pattern Recognition, pp 125-128. Tsukuba Science City, Japan. November 2012.
- [ICPR 2012] J.Mitra, Z.Kato, S.Ghose, D.Sidibé, R.Martí, X.Lladó, A.Oliver, J.C.Vilanova, and F.Meriaudeau. Spectral clustering to model deformations for fast multimodal registration. International Conference on Pattern Recognition, pp 2622-2625. Tsukuba Science City, Japan. November 2012.
- [ICIP 2012] J.Mitra, S.Ghose, D.Sidibé, A.Oliver, R.Martí, X.Lladó, J.C.Vilanova, J.Comet, and F.Meriaudeau. Weighted likelihood function of multiple statistical parameters to retrieve 2D TRUS-MR slice correspondence for prostate biopsy. IEEE International Conference on Image Processing, pp 2949-2952. Orlando, Florida. October 2012.
- [ICIP 2012] S.Ghose, J.Mitra, A.Oliver, R.Martí, X.Lladó, J.Freixenet, J.C.Vilanova, D.Sidibé, and F.Meriaudeau. A coupled schema of probabilistic atlas and statistical shape and appearance model for 3D prostate segmentation in MR images. IEEE International Conference on Image Processing, pp 541-544. Orlando, Florida. October 2012.
- [BP 2012] A.Oliver, J.Batlle, J.L.delaRosa, J.Salvi. Prova pilot: l'assignatura Estructura i Tecnologia de Computadors (grau d'Enginyeria Informàtica) dins la plataforma Bones Notes. Jornades de Bones Pràctiques, pp 1-6. Girona, Spain. September 2012.
- [BP 2012] R.Martí, A.Oliver, X.Lladó, X.Cufí, J.Martí, J.Freixenet, J.Salvi. VIBOT Day: un exemple de bones pràctiques universitat-empresa. Jornades de Bones Pràctiques, pp 1-5. Girona, Spain. September 2012.
- [ACIVS 2012] S.Ghose, J.Mitra, A.Oliver, R.Martí, X.Lladó, J.Freixenet, J.C.Vilanova, D.Sidibé, and F.Meriaudeau. A supervised learning framework for automatic prostate segmentation in transrectal ultrsound images. Advanced Concepts for Intelligent Vision Systems, LNCS 7517, pp 190-200. Brno, Czech Republic. September 2012.
- [EMBC 2012] J.Mitra, S.Ghose, D.Sidibé, R.Martí, A.Oliver, X.Lladó, J.C.Vilanova, J.Comet, and F.Meriaudeau. Joint probability of shape and image similarities to retrieve 2D TRUS-MR slice correspondence for prostate biopsy. IEEE Conference of the Engineering in Medicine and Biology Society, pp 5416-5419. San Diego, California. August 2012.
- [EMBC 2012] S.Ghose, J.Mitra, A.Oliver, R.Martí, X.Lladó, J.Freixenet, J.C.Vilanova, J.Comet, D.Sidibé, and F.Meriaudeau. Spectral clustering of shape and probability prior model for automatic prostate segmentation in ultrasound images. IEEE Conference of the Engineering in Medicine and Biology Society, pp. 2335-2338. San Diego, California. August 2012.
- [MIUA 2012] Z.Chen, A.Oliver, E.R.E.Denton, C.Boggis, and R.Zwiggelaar. Classification of microcalcification clusters using topological structure features. Medical Image Understanding and Analysis, pp 37-42. Swansea, Wales, UK. July 2012.
- [IWDM 2012] M.Tortajada, A.Oliver, R.Martí, M.Vilagran, S.Ganau, L.Tortajada, M.Sentís, and J.Freixenet. Adapting breast density classification from digitized to full-field digital mammograms. International Workshop on Digital Mammography, LNCS 7361, pp 561-568. Philadelphia, Pennsylvania. July 2012.
- [IWDM 2012] J.Massich, F.Meriaudeau, M.Sentís, S.Ganau, E.Pérez, R.Martí, A.Oliver, and J.Martí. Automatic seed placement for breast lesion segmentation on US images. International Workshop on Digital Mammography, LNCS 7361, pp 308-315. Philadelphia, Pennsylvania. July 2012.
- [SPIE 2012] J.Mitra, A.Srikantha, D.Sidibé, R.Martí, A.Oliver, X.Lladó, S.Ghose, J.C.Vilanova, J.Comet, and F.Meriaudeau. A shape-based statistical method to retrieve 2D TRUS-MR slice correspondence for prostate biopsy. SPIE Conference on Medical Imaging, Proc. SPIE 8314, pp 83143M1-83143M9. San Diego, California. February 2012.
- [SPIE 2012] S.Ghose, A.Oliver, R.Martí, X.Lladó, J.Freixenet, J.Mitra, J.C.Vilanova, J.Comet, and F.Meriaudeau. A hybrid framework of multiple active appearance models and global registration for 3D prostate segmentation in MRI. SPIE Conference on Medical Imaging, Proc. SPIE 8314, pp 83140S1-83140S9. San Diego, California. February 2012.
- [DICTA 2011] J.Mitra, Z.Kato, R.Martí, A.Oliver, X.Lladó, S.Ghose, J.C.Vilanova, and F.Meriaudeau. A non-linear diffeomorphic framework for prostate multimodal registration. International Conference on Digital Image Computing: Techniques and Applications, pp 31-36. Noosa, Australia. December 2011.
- [DICTA 2011] S.Ghose, A.Oliver, R.Martí, X.Lladó, J.Freixenet, J.Mitra, J.C.Vilanova, J.Comet, and F.Meriaudeau. Statistical shape and probability prior model for automatic prostate segmentation. International Conference on Digital Image Computing: Techniques and Applications, pp 340-345. Noosa, Australia. December 2011.
- [MICCAI-PCI 2011] S.Ghose, A.Oliver, R.Martí, X.Lladó, J.Freixenet, J.Mitra, J.C.Vilanova, J.Comet, and F.Meriaudeau. Multiple mean models of statistical shape and probability priors for automatic prostate segmentation. MICCAI Workshop on Prostate Cancer Imaging: Computer Aided Diagnosis, Prognosis, and Intervention, LNCS 6963, pp 35-46. Toronto, Canada. September 2011.
- [MICCAI-BIA 2011] J.Massich, F.Meriaudeau, E.Pérez, R.Martí, A.Oliver, and J.Martí. Seed selection criteria for breast lesion segmentation in ultra-sound images. MICCAI Workshop on Breast Image Analysis, pp 55-64. Toronto, Canada. September 2011.
- [ICIP 2011] S.Ghose, A.Oliver, R.Martí, X.Lladó, J.Freixenet, J.C.Vilanova, and F.Meriaudeau. A probabilistic framework for automatic prostate segmentation with a statistical model of shape and appearance. IEEE International Conference on Image Processing, pp 725-728. Brussels, Belgium. September 2011.
- [ICIP 2011] M.Peracaula, A.Oliver, A.Torrent, X.Lladó, J.Freixenet, and J.Martí. Segmenting extended structures in radio astronomical images by filtering brigth compact sources and using wavelets decomposition. IEEE International Conference on Image Processing, pp 2861-2864. Brussels, Belgium. September 2011.
- [EDULEARN 2011] J.Martí, C.Mata, X.Lladó, and A.Oliver. MamoDB: a web-based tool for training radiologists in the diagnosis of digital mammography. International Conference on Education and New Learning Technologies, pp 2359-2367. Barcelona, Spain. July 2011.
- [SPIE 2011] J.Mitra, R.Martí, A.Oliver, X.Lladó, J.C.Vilanova, and F.Meriaudeau. A comparison of thin-plate splines with automatic correspondences and b-splines with uniform grids for multimodal prostate registration. SPIE Conference on Medical Imaging, Proc. SPIE 7964, pp 79642T1-79642T8. Orlando, Florida. February 2011.
- [SPIE 2011] S.Ghose, A.Oliver, R.Martí, X.Lladó, J.Freixenet, J.C.Vilanova, and F.Meriaudeau. Prostate segmentation with local binary patterns guided active appearance model. SPIE Conference on Medical Imaging, Proc. SPIE 7962, pp 7962181-7962188. Orlando, Florida. February 2011.
- [SITIS 2010] J.Mitra, A.Oliver, R.Martí, X.Lladó, J.C.Vilanova, and F.Meriaudeau. A thin-plate spline based multimodal prostate registration with optimal correspondences. International Conference on Signal-Image Technology & Internet-Based Systems, pp 7-11. Kuala Lumpur, Malaysia. December 2010.
- [SITIS 2010] S.Ghose, A.Oliver, R.Martí, X.Lladó, J.Freixenet, J.C.Vilanova, and F.Meriaudeau. Prostate segmentation with texture enhanced Active Appearance Model. International Conference on Signal-Image Technology & Internet-Based Systems, pp 18-22. Kuala Lumpur, Malaysia. December 2010.
- [DICTA 2010] J.Mitra, A.Oliver, R.Martí, X.Lladó, J.C.Vilanova, and F.Meriadeau. Multimodal prostate registration using thin-plate splines from automatic correspondences. International Conference on Digital Image Computing: Techniques and Applications, pp 587-592. Sydney, Australia. December 2010.
- [ADASS 2010] M.Peracaula, X.Lladó, J.Freixenet, A.Oliver, A.Torrent, J.M.Paredes, and J.Martí. Segmentation and detection of extended structures in low frequency astronomical surveys using hybrid wavelet decomposition. International Conference on Astronomical Data Analysis Software and Systems, ASP Conference Series 442, pp 151-154. Boston, Massachusetts. November 2010.
- [CASEIB 2010] C.Mata, X.Lladó, R.Martí, J.Freixenet, A.Oliver, and J.Martí. Aplicación web para la gestión de una base de datos pública en mamografía digital: MamoDB. Congreso Anual de la Sociedad Española de Ingeniería Biomédica, pp 175-178. Madrid, Spain. November 2010.
- [ICIP 2010] A.Torrent, A.Oliver, X.Lladó, R.Martí, and J.Freixenet. A supervised micro-calcification detection approach in digitised mammograms. IEEE International Conference on Image Processing, pp 4345-4348. Hong Kong. September 2010.
- [ICIP 2010] Y.Díez, A.Oliver, X.Lladó, and R.Martí. Comparison of registration methods using mammographic images. IEEE International Conference on Image Processing, pp 4421-4424. Hong Kong. September 2010.
- [MICCAI-PCI 2010] S.Ghose, A.Oliver, R.Martí, X.Lladó, J.Freixenet, J.C.Vilanova, and F.Meriaudeau. Texture guided Active Appearance Model propagation for prostate segmentation. MICCAI Workshop on Prostate Cancer Imaging: Computer Aided Diagnosis, Prognosis, and Intervention, LNCS 6367, pp 111-120. Beijing, China. September 2010.
- [EMBC 2010] M.Tortajada, A.Oliver, Y.Díez, R.Martí, J.C.Vilanova, and J.Freixenet. Improving a CAD system using bilateral information. IEEE Conference of the Engineering in Medicine and Biology Society, pp 5054-5057. Buenos Aires, Argentina. September 2010.
- [ICPR 2010] A.Oliver, A.Torrent, X.Lladó, and J.Martí. Automatic diagnosis of masses by using level set segmentation and shape description. International Conference on Pattern Recognition, pp 2528-2531. Istanbul, Turkey. August 2010.
- [IWDM 2010] A.Oliver, A.Torrent, M.Tortajada, X.Lladó, M.Peracaula, L.Tortajada, M.Sentís, and J.Freixenet. A boosting based approach for automatic micro-calcification detection. International Workshop on Digital Mammography, LNCS 6136, pp 251-258. Girona, Spain. June 2010.
- [IWDM 2010] J.Massich, F.Meriaudeau, E.Pérez, R.Martí, A.Oliver, and J.Martí. Lesion segmentation in breast sonography. International Workshop on Digital Mammography, LNCS 6136, pp 39-45. Girona, Spain. June 2010.
- [IWDM 2008] A.Torrent, A.Bardera, A.Oliver, J.Freixenet, I.Boada, M.Feixas, R.Martí, X.Lladó, J.Pont, E.Pérez, S.Pedraza, and J.Martí. Breast density segmentation: a comparison of clustering and region based techniques. International Workshop on Digital Mammography, LNCS 5116, pp 9-16. Tucson, Arizona. July 2008.
- [UNIVEST 2008] X.Lladó, J.Freixenet, and A.Oliver. How we designed a succesfull ECTS Master's Degree course in a multicultural environment?. International Conference UNIVEST. Girona, Spain. June 2008.
- [MICCAI 2007] A.Oliver, X.Lladó, J.Freixenet, and J.Martí. False Positive Reduction in Mammographic Mass Detection using Local Binary Patterns. International Conference on Medical Image Computing and Computer Assisted Intervention, LNCS 4791, pp 286-293. Brisbane, Australia. October 2007.
- [MIUA 2007] A.Oliver, X.Lladó, R.Martí, J.Freixenet, and R.Zwiggelaar. Classifying mammograms using texture information. Medical Image Understanding and Analysis, pp 223-227. Aberystwyth, Wales, UK. July 2007.
- [MIUA 2007] X.Lladó, A.Oliver, J.Martí, and J.Freixenet. Dealing with false positive reduction in mammographic mass detection. Medical Image Understanding and Analysis, pp 81-85. Aberystwyth, Wales, UK. July 2007. (Best Poster Prize)
- [IbPRIA 2007] A.Oliver, X.Lladó, J.Martí, R.Martí, and J.Freixenet. False Positive Reduction in Breast Mass Detection using Two-Dimensional PCA. Iberian Conference on Pattern Recognition and Image Analysis, LNCS 4478, pp 154-161. Girona, Spain. June 2007.
- [IbPRIA 2007] R.Martí, A.Oliver, D.Raba, and J.Freixenet. Breast skin-line segmentation using contour growing. Iberian Conference on Pattern Recognition and Image Analysis, LNCS 4478, pp 564-571. Girona, Spain. June 2007.
- [MICCAI 2006] A.Oliver, J.Freixenet, R.Martí, and R.Zwiggelaar. A comparison of breast tissue classification techniques. International Conference on Medical Image Computing and Computer Assisted Intervention, LNCS 4191, pp 872-879. Copenhagen, Denmark. October 2006.
- [ICPR 2006] A.Oliver, J.Martí, R.Martí, A.Bosch, and J.Freixenet. A new approach to the classification of mammographic masses and normal breast tissue. International Conference on Pattern Recognition, vol 4 pp 707-710. Hong Kong. August 2006.
- [ICPR 2006] A.Bosch, X.Muñoz, A.Oliver, and R.Martí. Object and scene classification: what does a supervised approach provide us?. International Conference on Pattern Recognition, vol 1 pp 773-777. Hong Kong. August 2006.
- [MIUA 2006] A.Oliver, J.Freixenet, R.Martí, E.R.E.Denton, and R.Zwiggelaar. Mammographic mass eigendetection. Medical Image Understanding and Analysis, pp 71-75. Manchester, UK. July 2006.
- [IWDM 2006] I.Muhimmah, A.Oliver, E.R.E.Denton, J.Pont, E.Pérez, and R.Zwiggelaar. Comparison between Wolfe, Boyd, BI-RADS and Tabár based Mammographic Risk Assessment. International Workshop on Digital Mammography, LNCS 4046, pp 407-415. Manchester, UK. June 2006.
- [IWDM 2006] R.Martí, D.Raba, A.Oliver, and R.Zwiggelaar. Mammographic Registration: Proposal and Evaluation of a New Approach. International Workshop on Digital Mammography, LNCS 4046, pp 213-220. Manchester, UK. June 2006.
- [CVPR 2006] A.Bosch, X.Muñoz, A.Oliver, and J.Martí. Modeling and Classifying Breast Tissue Density in Mammograms. IEEE Conference on Computer Vision and Pattern Recognition, vol 2 pp 1552-1558. New York. June 2006.
- [AQTR 2006] A.Oliver, X.Muñoz, J.Batlle, Ll.Pacheco, and J.Freixenet. Improving clustering algorithms for image segmentation using contour and region information. IEEE-TTTC International Conference on Automation, Quality & Testing, Robotics, pp 315-320. Cluj-Napoca, Romania. May 2006.
- [CCIA 2005] A.Oliver, J.Freixenet, M.Peracaula, and J.Martí. Mass Segmentation using a Pattern Matching Approach with a Mutual Information based Metric. Congrés Català d'Intel·ligència Artificial, pp 123-130. L'Alguer, Italy. October 2005.
- [CCIA 2005] A.Bosch, X.Muñoz, J.Martí, and A.Oliver. Classifying Natural Objects on Outdoor Scenes. Congrés Català d'Intel·ligència Artificial, pp 115-122. L'Alguer, Italy. October 2005.
- [ICIP 2005] A.Oliver, J.Freixenet, and R.Zwiggelaar. Automatic Classification of Breast Density. IEEE International Conference on Image Processing, vol 2 pp 1258-1261. Genova, Italy. September 2005.
- [IbPRIA 2005] A.Oliver, J.Freixenet, A.Bosch, D.Raba, and R.Zwiggelaar. Automatic Classification of Breast Tissue. Iberian Conference on Pattern Recognition and Image Analysis, LNCS 3523, pp 431-438. Estoril, Portugal. June 2005.
- [IbPRIA 2005] D.Raba, A.Oliver, J.Martí, M.Peracaula, and J.Espunya. Breast Segmentation with Pectoral Muscle Suppression on Digital Mammograms. Iberian Conference on Pattern Recognition and Image Analysis, LNCS 3523, pp 471-478. Estoril, Portugal. June 2005.
- [BMVC 2003] X.Lladó, A.Oliver, M.Petrou, J.Freixenet, and J.Martí. Simultaneous Surface Texture Classification and Illumination Tilt Angle Prediction. British Machine Vision Conference, pp 789-798. Norwich, UK. September 2003.
- [IbPRIA 2003] J.Martí, J.Freixenet, X.Muñoz, and A.Oliver. Active Region Segmentation of Mammographic Masses based on Texture, Contour and Shape Features. Iberian Conference on Pattern Recognition and Image Analysis, LNCS 2652, pp 478-485. Mallorca, Spain. June 2003.
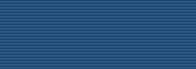
Abstracts in Conferences
- [ISBI 2024] A.Casamitjana, A.Oliver, X.Lladó. Anatomically-aware automatic quantification of DaTSCAN images. IEEE International Symposium on Biomedical Imaging. Athens, Greece. May 2024.
- [ISMRM 2022] Ll.Coll, P.Carbonell-Mirabent, Á.Cobo-Calvo, G.Arrambide, A.Vidal-Jordana, M.Comabella, J.Castilló, B.Rodríguez-Acebedo, A.Zabalza, I.Galán, L.Midaglia, C.Nos, A.Salerno, C.Auger, M.Alberich, J.Río, J.Sastre-Garriga, A.Oliver, X.Montalban, À.Rovira, M.Tintoré, D.Pareto, X.Lladó, and C.Tur. Classification of MS patients into disability levels using deep learning approaches based solely on routinely-acquired MRI. Annual Meeting of the International Society for Magnetic Resonance in Medicine. London, UK. May 2022.
- [SEN 2021] J.F.Corral, J.Alonso, C.Auger, S.Valverde, A.Vidal-Jordana, A.Oliver, A.Barros, K.Ng Wong, M.Tintoré, F.X.Aymerich, X.Montalban, X.Lladó, and A. Rovira. Evaluación de sistemas automáticos para la detección de lesiones T2 en pacientes con esclerosis múltiple. Congreso de la Sociedad Española de Neurología. Online event, Spain. November 2021.
- [SERAM 2021] S.Valverde, A.Oliver, and X.Lladó. Aplicaciones automáticas de soporte al neurólogo para mejorar la sensibilidad ante la presencia de lesiones nuevas en T2 en esclerosis múltiple. Congreso de la Sociedad Española de Radiología. Madrid, Spain. May 2021.
- [SERAM 2018] S.Valverde, A.Oliver, J.C.Vilanova, Ll.Ramió-Torrentà, À.Rovira, and X.Lladó. Herramientas basadas en deep learning para la segmentación de imágenes de RM. Aplicación a la cuantificación de lesiones de esclerosis múltiple. Congreso de la Sociedad Española de Radiología. Pamplona, Spain. May 2018.
- [ESMRMB 2017] C.Mata, P.Walker, A.Lalande, J.Martí, and A.Oliver. Benefits of a collaborative work in the evaluation of prostate cancer from MRI. Annual Scientific Meeting of the European Society for Magnetic Resonance in Medicine and Biology. Barcelona, Spain. October 2017.
- [SERAM 2016] M.Cabezas, A.Oliver, X.Lladó, D.Pareto, and À.Rovira. Análisis del uso de la imagen de sustracción para detectar nuevas lesions de T2 en pacientes de esclerosis múltiple. Congreso de la Sociedad Española de Radiología. Bilbao, Spain. May 2016.
- [ASNR 2015] M.Cabezas, A.Oliver, X.Lladó, D.Pareto, and À.Rovira. A pipeline for detecting new multiple sclerosis lesions on longitudinal brain magnetic resonance imaging. American Society of NeuroRadiology Annual Meeting. Chicago, Illinois. April 2015.
- [ECR 2013] M.Tortajada, A.Oliver, Y.Díez, J.C.Vilanova, J.Martí, J.Freixenet, S.Ganau, L.Tortajada, R.Aguilar, M.Vives, J.Fernandez-Bayo, M.Sentís, and R.Martí. CAD system using case-based information. Annual Meeting of the European Society of Radiology. Vienna, Austria. March 2013.
- [ESMRMB 2012] C.Mata, P.Walker, A.Lalande, F.Brunotte, A.Oliver, and J.Martí. ProstateAnalyzer: GUI in medical domain with management of DICOM images of prostate cancer. Annual Scientific Meeting of the European Society for Magnetic Resonance in Medicine and Biology. Lisboa, Portugal. October 2012.
- [RSNA 2010] M.Tortajada, A.Oliver, Y.Díez, R.Martí, J.C.Vilanova, and J.Freixenet. Integrating bilateral information in the eigendetection CAD approach. Scientific Assembly and Annual Meeting of the Radiological Society of North America. Chicago, Illinois. December 2010.
- [CARS 2005] A.Oliver, J.Martí, J.Freixenet, J.Pont, and R.Zwiggelaar. Automatic classification of breast density according BIRADS categories using a clustering approach. Computer Assisted Radiology and Surgery International Congress and Exhibition. Berlin, Germany. June 2005.
- [CARS 2005] J.Freixenet, D.Raba, A.Oliver, and J.Espunya. Breast profile segmentation based on the region growing approach. Computer Assisted Radiology and Surgery International Congress and Exhibition. Berlin, Germany. June 2005.
- [IWDM 2004] J.Martí, J.Freixenet, M.Peracaula, A.Oliver, D.Raba, J.Espunya, J.Pont, and R.Martí. Automatic segmentation of microcalcifications based on the fusion of different algorithms over CC and MLO views. International Workshop on Digital Mammography. Chapel Hill, North Carolina. June 2004.
- [SERAM 2004] A.Oliver, J.Freixenet, J.Martí, and R.Zwiggelaar. Estudio comparativo de cuatro métodos para la detección automática de masas en mamografías digitales. Congreso de la Sociedad Española de Radiología. Bilbao, Spain. May 2004.
- [CCSPM 2004] J.Pont, J.Martí, R.Bassaganyas, D.Raba, R.Martí, A.Oliver, M.Peracaula, J.Espunya, E.Golobardes, and J.Freixenet. Cerca de documentació complementària en bases de dades de mamografies digitals per ajudar en el diagnòstic precoç. Congrés Català de Sinologia i Patologia Mamària. Girona, Catalonia. May 2004.
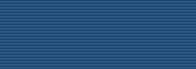